20 Lessons for the Developing Player by John Novak & Radek Dobias (2013)
Backgammon Magic is a slight book of 20 “lessons” – interesting positions with analysis – and a potentially interesting idea, which is that neural-net analysis renders many classic backgammon “rules” incorrect. There is a lot of emphasis on play against AI (and some dismissal of the advice and rules of thumb of backgammon greats), and all the problems purely concern checker play.
Interesting idea but major flaw in analysis
Many of the principles make sense and the lessons may be useful for a beginner. I am a thoroughly intermediate player and had not much trouble finding the “hidden” plays: I am somewhat sceptical that there would be much for expert players that is not better covered elsewhere.
Needless to say, the validity of pretty much the whole book rests upon the accuracy of the bot evaluation of the positions. Unfortunately, there seems to be a serious flaw in the analysis methodology.
The strangest match score
As the author (helpfully) indicates in the introduction, all analysis was performed in GNUBG at cubeful 3-ply – and here’s the important bit – on 1-point matches. A 1-point match is equivalent to double match-point (DMP), one of the most distorted backgammon scores there is. At DMP both gammons and backgammons are worth nothing extra, and the cube is also dead. This can dramatically affect correct checker play.
Nobody seriously plays 1-point matches. It seemed to be the authors’ intention to eliminate all consideration of the cube from the problems, but by going straight to DMP they have ironically selected the most score-dependent situation you can get: really they wanted to evaluate the positions as money games (without Jacoby) or as 0-0 in a long match (which approximates to the same).
Some Examples
This isn’t just of academic interest. I analysed several of the positions in GNU, directly and by rollout – and while I found (roughly) the same results at 1-away 1-away, adjusting to money or the first game in a long (15 point) match frequently changed the predicted “best” move.
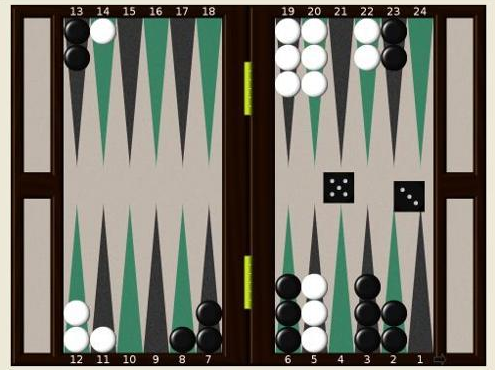
Position #20 is particularly wrong here. The recommendation is to run from a back anchor into a triple shot “simply because there is nothing better”. But white has a triple shot and another direct shot, a three-point board, and lots of builders poised to close. Even to an intermediate player this looks bad: it’s too gammonish. A rollout at money or in a long match indicates that the 13/10 13/8 is in fact better, and that 23/15 loses a huge number of gammons (25% compared to 17%) and double the number of backgammons, even as it wins slightly more games.
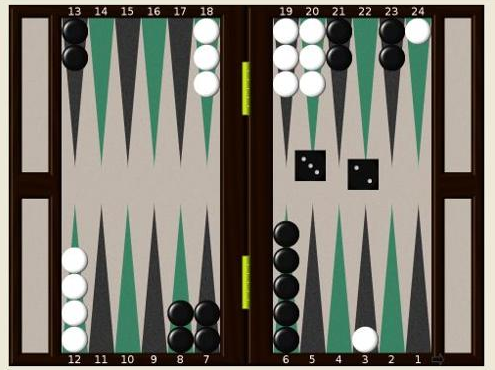
Position #11 (lesson: “have balls”) suffers in a similar way. The recommendation is to hit loose in board and slot another board point – basically a “banana split” (the advice is “sometimes you must do what you feel the least like doing”). The problem is that, by eliminating consideration of gammons and the cube, they don’t properly take into account the volatility of such a big play. A rollout indicates that actually the “bad” move 13/11 6/3* is best, precisely because it loses less gammons (21.1% vs 21.8%) even if it wins slightly less games. The actual equity differences are in fact very small, so it’s not really clear what there is to learn from this position.
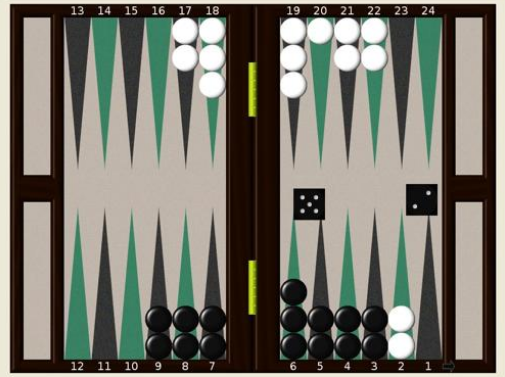
Having a dead cube can sometimes make whole evaluations unnecessary. In position #18, clearing the 8 point is indeed best at DMP. But at any score with a live cube, GNU indicates that all the plausible moves evaluate the same. Why? Because white has a killer double/pass and the chance of gammon is negligible. At any normal score, this would be a claim.
Less seriously, their evaluations are a bit superficial. Having selected the positions, why didn’t they do a 4-ply evaluation (which takes just a few minutes longer?) – or better, roll them out? Why didn’t they use XG, when they identify it as the best bot? (maybe they’re a stingy as me).
Over-confident
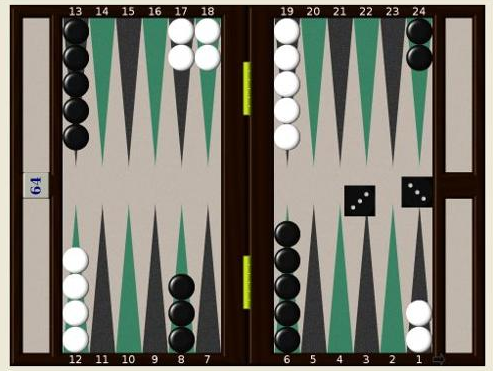
There is some rather strident advice in position #1 about the best move in the very first move of the game. Unfortunately, when you roll it out, the equity difference becomes rather less than reported (the “bad” move is -0.69% game winning chance, down from an already tiny -1.01%). And away from DMP the effect disappears completely: in a rollout in a 15-point match, the equity difference between the three reported moves then becomes smaller than 0.1% MWC with a standard deviation of 0.06% – statistically the moves are indistinguishable, and indeed unstacking the midpoint is no longer best. The position, as might be expected so early in the game, is simply a wash: there is nothing to justify the strong conclusion that “sorry, but [the idea that making the 5 point is best] is simply not true … an expert will unstack. The intermediate player will make the 5 point”.
Aside from this, the authors can come across as a little self-aggrandising, and this can grate. You probably need to start winning some actual tournaments before you compare yourself to Nack Ballard.
Aside from this, the author(s) can come across as a little self-aggrandising, and this can grate. You probably need to start winning some actual tournaments before you compare yourself to Nack Ballard.
There is a sense that they haven’t read too deeply into the backgammon “literature” (as they describe it): where their advice is good, it’s fairly easy to find equivalent advice, often from 10-20 years ago. Their “best backgammon advice” is indeed great: “if you don’t see it, you can’t play it” – but is straight out of Kit Woolsey, who in turn got it from none other than Paul Magriel. Some of the tips for improving are also very odd: the idea that IQ improving exercises can do anything for your backgammon doesn’t even seem to be supported by anecdote.
There is some good stuff in here – and I like the idea of finding truly counter-intuitive plays recommended by the AI: these do exist, and with large equity swings that are not just noise in the evaluations. A beginner can likely benefit from this book, but anyone beyond will probably find it a more interesting exercise in how DMP varies from normal match play.